Building the future in robust, interpretable, and scalable solutions for distributed machine learning problems.
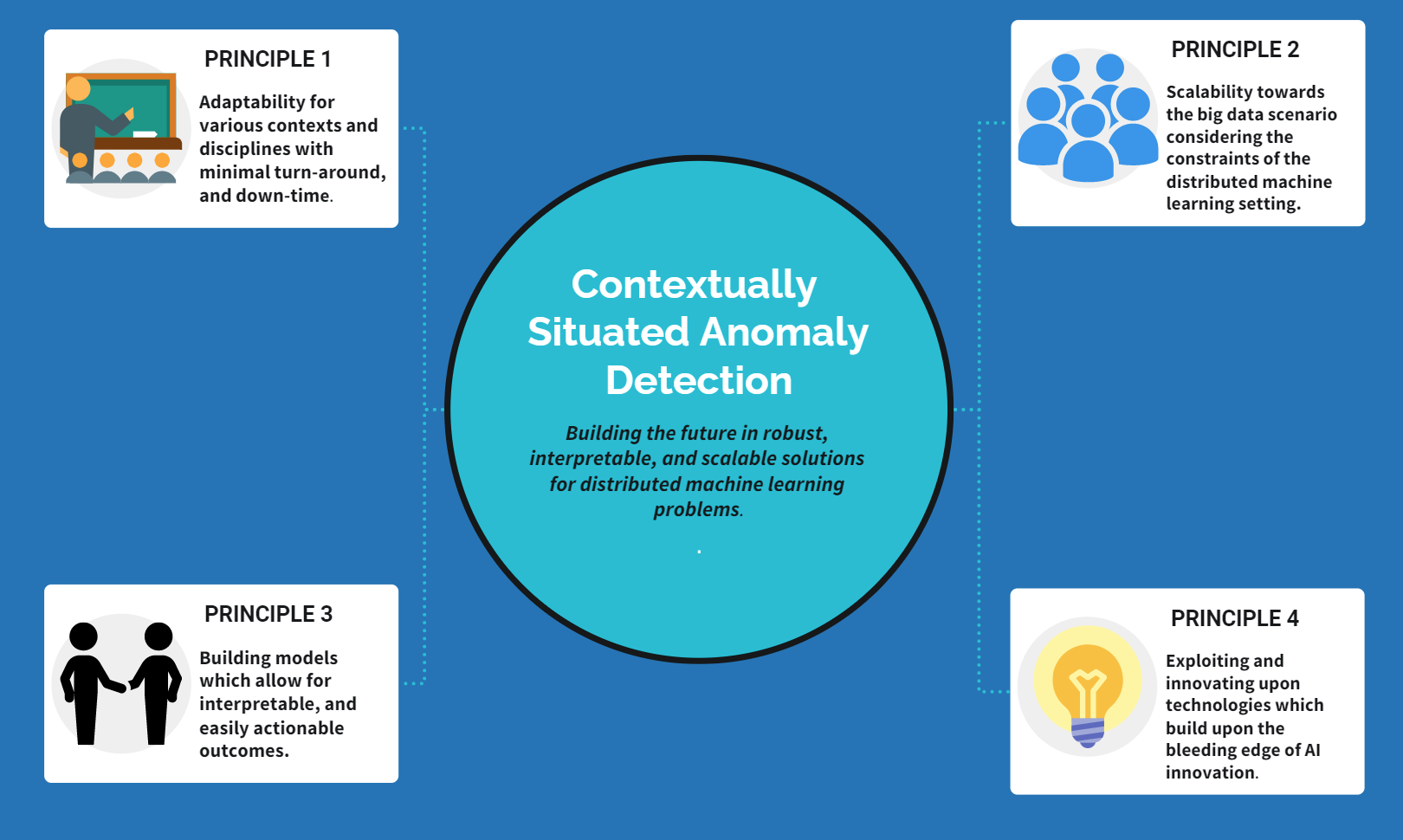
Our story
The use of sensor technologies underpins everyday life for Australians in many ways; ranging from wearable medical devices, all the way to localized smart home network devices. Such sensors tend to work in a distributed setting, whether in their own local network, or over the cloud. Thus, there is an urgent call to action when it comes developing solutions for the efficacy of sensor array networks for the intelligent monitoring of damage detection, damage localization, and context characterization. As a team, we have collectively recognized that there is a need to push for bleeding edge developments in the space of structural health monitoring, and aim to provide compelling, and interpretable solutions in this space.
Our mission
- We are a solutions-oriented team, focused on solving the many outstanding problems that currently exist in the structural health monitoring field.
- We aim to innovate several technologies within Australia for the distributed sensor problem that focus on time series applications.
- We are collaborative in nature, and welcome the creation of new value, especially when we can convert theoretical intuitions into experimental success.
Our philosophy
We are motivated by a four-point design philosophy that focuses on the principles of adaptability, scalability, interpretability, and bleeding-edge innovation when comes to solving distributed learning problems which focus on contextually situated anomaly detection problems.